Stay up to date with our latest case studies, thought pieces and news, delivering insights you can really use.
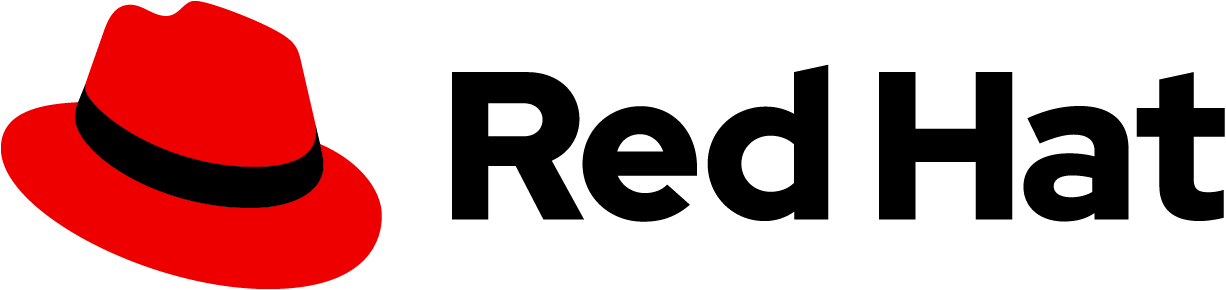
Red Hat® AAP 1.2 (Ansible Tower 3.8) Support Life Cycle Reminder
The support life cycle for Red Hat® Ansible® Automation Platform 1.2 (Ansible Tower 3.8) ends September 29, 2023 per the posted life cycle policy. To avoid potential maintenance, bug, and security complications, customers will need to migrate to the latest version of Red Hat Ansible Automation Platform 2 before September 29, 2023.
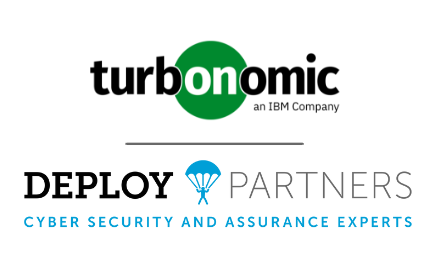
Optimising Application Performance with Turbonomic ARM: The Benefits of Automated Resource Management
Discover how Turbonomic ARM can help optimise your IT infrastructure and improve the performance of your applications.
DeployPartners Sponsor Monika’s DoggieRescue
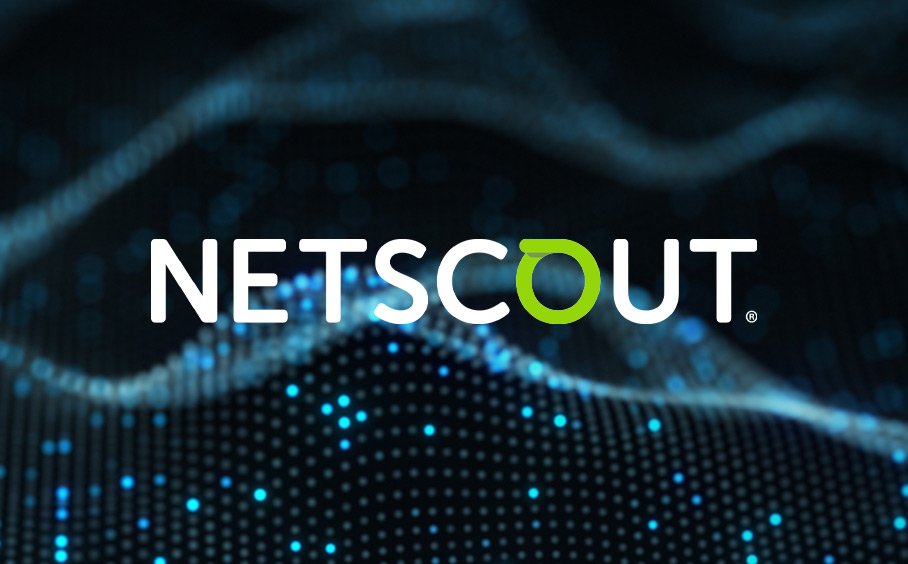
DeployPartners expands NetScout TrueCall at Spark NZ
DeployPartners were engaged to expand the NetScout TrueCall RAN Geoanalytics platform at Spark, a major New Zealand teleco provider.
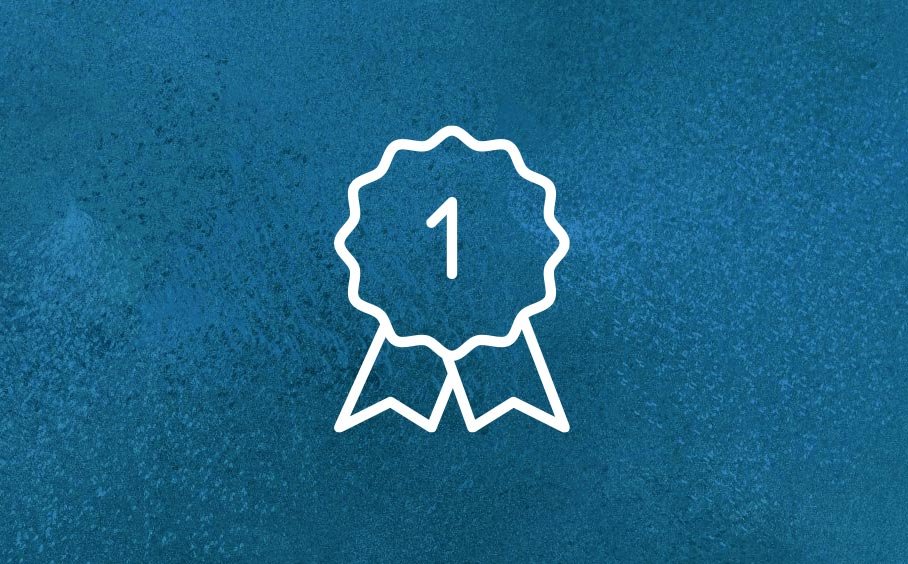
DeployPartners named Red Hat’s ‘New Partner of the Year’ for 2022
DeployPartners are extremely proud to be recognised as 'New Partner of the Year' at the annual Red Hat Australia and New Zealand Partner Awards.
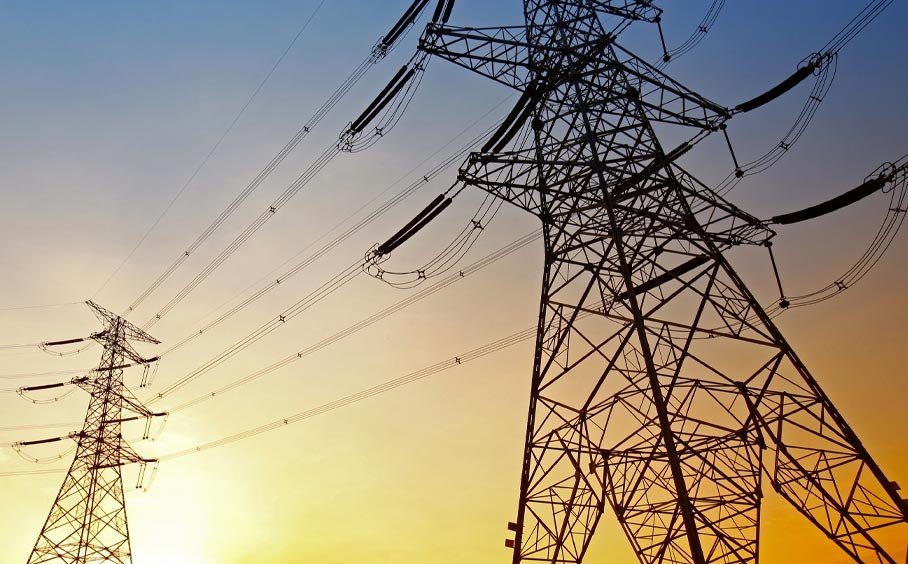
DeployPartners helps to maintain software currency at TasNetworks
See how DeployPartners provided TasNetworks with a solution to maintain software currency with minimal impact on existing systems and operations.
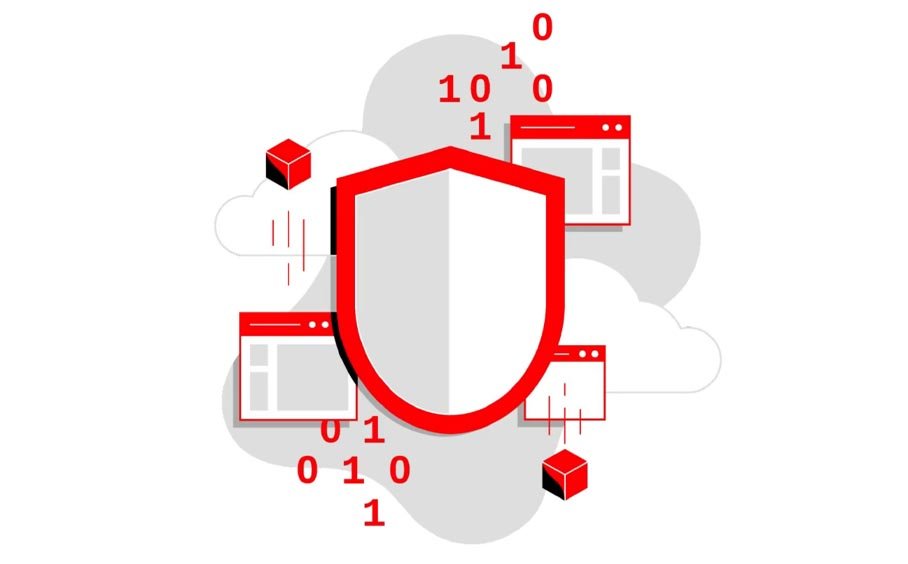
Cyber Security with Red Hat and DeployPartners
See how Australian organisations automate their cyber security in this on-demand webinar.
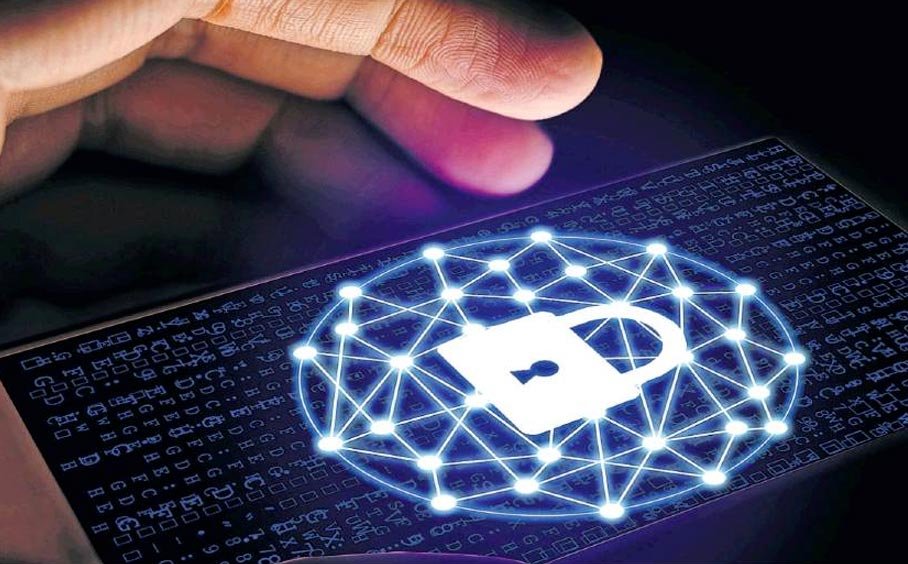
“Cyber complexity is the real enemy” - AFR piece
DeployPartners comment on the state of Cyber Security in this Australian Finance Review article.
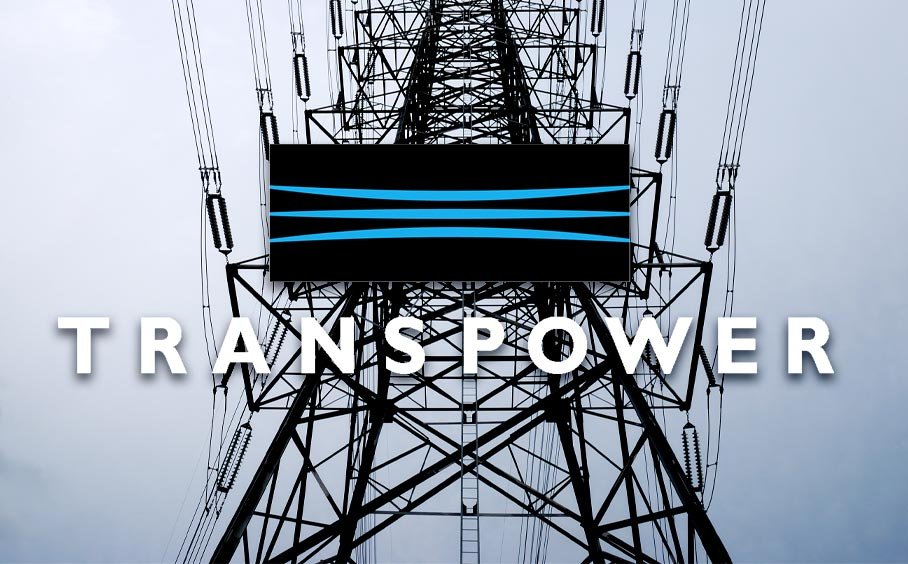
Transpower NZ selects DeployPartners
Transpower selects DeployPartners to manage their IT Operations Fault Management platform.